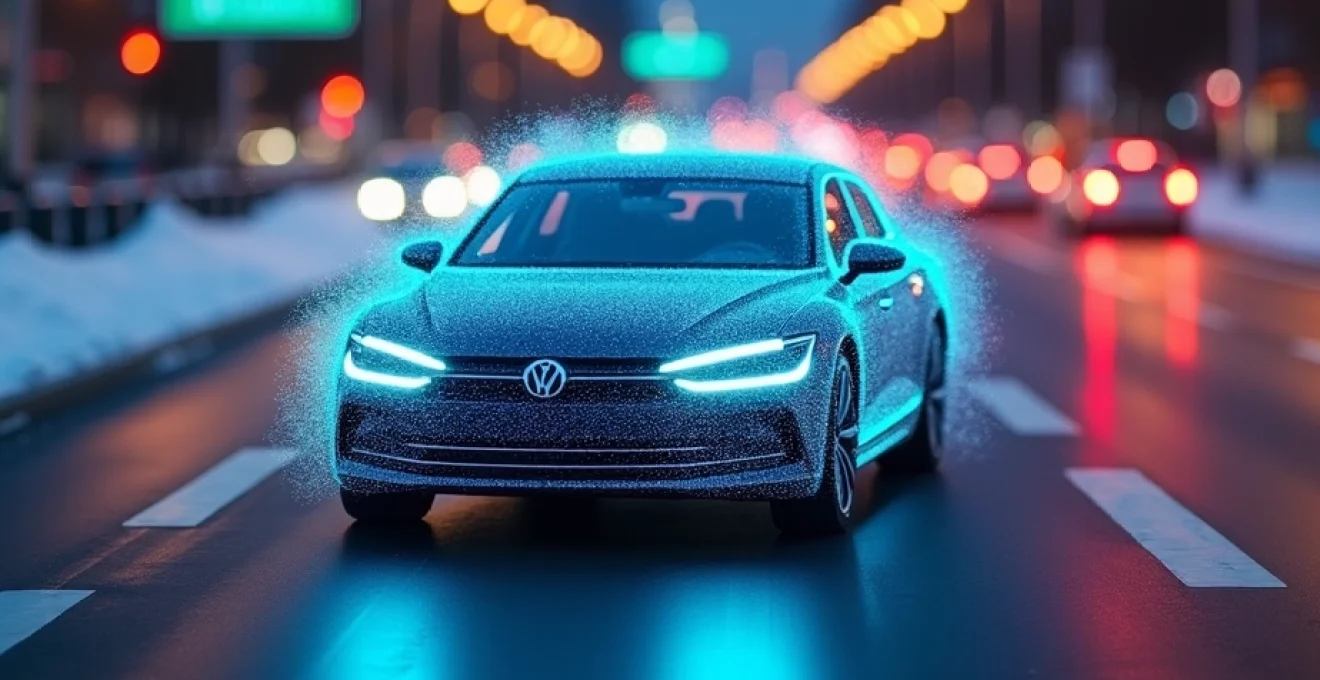
Autonomous driving technology is poised to revolutionize transportation, promising enhanced safety and improved efficiency on our roads. As vehicles become increasingly intelligent and interconnected, the potential for reducing accidents, optimizing traffic flow, and transforming urban landscapes grows exponentially. This shift towards self-driving cars represents not just a technological leap, but a fundamental reimagining of how we interact with our vehicles and transportation infrastructure.
Machine learning algorithms powering autonomous vehicle decision-making
At the heart of autonomous driving technology lies a sophisticated network of machine learning algorithms that enable vehicles to perceive their environment, make split-second decisions, and navigate complex traffic scenarios. These algorithms form the cognitive backbone of self-driving cars, allowing them to process vast amounts of sensor data and react to dynamic road conditions with superhuman speed and precision.Deep neural networks for real-time object detection
Deep neural networks (DNNs) play a crucial role in autonomous vehicles' ability to identify and classify objects in their surroundings. These artificial intelligence systems are trained on millions of images to recognize everything from pedestrians and cyclists to traffic signs and road markings. By employing convolutional neural networks (CNNs), self-driving cars can achieve high-accuracy object detection in real-time, even under challenging lighting and weather conditions.Reinforcement learning in dynamic traffic scenarios
Reinforcement learning algorithms enable autonomous vehicles to adapt to complex and unpredictable traffic situations. By simulating countless driving scenarios, these systems learn optimal decision-making strategies through trial and error. This approach allows self-driving cars to navigate intricate urban environments, anticipate the actions of other road users, and make safe, efficient choices in real-world driving conditions.Sensor fusion techniques for environmental perception
Autonomous vehicles rely on a diverse array of sensors to build a comprehensive picture of their surroundings. Sensor fusion algorithms combine data from multiple sources, including cameras, LiDAR, radar, and ultrasonic sensors, to create a robust and accurate representation of the environment. This multi-modal approach enhances the vehicle's ability to detect and track objects, estimate distances, and identify potential hazards with greater reliability than any single sensor type alone.Predictive modeling for anticipatory driving behavior
Advanced predictive modeling techniques allow autonomous vehicles to anticipate the future states of their environment and other road users. By analyzing historical data and current traffic patterns, these algorithms can forecast potential scenarios and plan optimal trajectories. This proactive approach to driving enables self-driving cars to make smoother, more efficient maneuvers and reduce the likelihood of accidents caused by sudden changes in traffic conditions.Advanced sensor technologies enhancing road safety
The safety and effectiveness of autonomous vehicles hinge on their ability to accurately perceive and interpret the world around them. A suite of cutting-edge sensor technologies works in concert to provide self-driving cars with a comprehensive understanding of their environment, far surpassing the capabilities of human drivers.Lidar systems for high-resolution 3D mapping
Light Detection and Ranging (LiDAR) technology serves as the eyes of many autonomous vehicles, creating detailed 3D maps of the surrounding area. By emitting laser pulses and measuring their reflections, LiDAR systems can generate highly accurate point clouds that represent the physical world with centimeter-level precision. This enables self-driving cars to navigate with exceptional accuracy, even in complex urban environments or areas with limited visibility.Millimeter-wave radar for all-weather perception
While LiDAR excels in ideal conditions, millimeter-wave radar provides crucial sensing capabilities in adverse weather. These systems can penetrate through fog, rain, and snow, allowing autonomous vehicles to maintain situational awareness in conditions that would severely impair human drivers. The ability to detect and track objects at long ranges makes radar an essential component of all-weather autonomous driving systems.Computer vision algorithms for traffic sign recognition
Advanced computer vision algorithms enable self-driving cars to interpret visual information from their surroundings, including the critical task of recognizing and understanding traffic signs and signals. By employing deep learning techniques, these systems can accurately identify and classify a wide variety of road signs, traffic lights, and lane markings, ensuring that autonomous vehicles can navigate in compliance with local traffic regulations.Ultrasonic sensors for close-range obstacle detection
For precise maneuvering in tight spaces, autonomous vehicles rely on ultrasonic sensors. These devices emit high-frequency sound waves and measure their echoes to detect nearby obstacles with high accuracy. Ultrasonic sensors are particularly useful for parking assistance and low-speed navigation in congested areas, complementing the longer-range capabilities of LiDAR and radar systems.V2X communication protocols for coordinated traffic flow
Vehicle-to-Everything (V2X) communication represents a paradigm shift in how vehicles interact with each other and their environment. By enabling real-time data exchange between cars, infrastructure, and other road users, V2X technology paves the way for unprecedented levels of traffic coordination and safety.DSRC vs. C-V2X standards for Vehicle-to-Vehicle communication
Two primary standards have emerged for V2X communication: Dedicated Short-Range Communications (DSRC) and Cellular Vehicle-to-Everything (C-V2X). DSRC operates on a dedicated 5.9 GHz band, offering low-latency communication ideal for time-critical safety applications. C-V2X, on the other hand, leverages existing cellular networks and promises broader coverage and integration with 5G technology. The industry continues to debate the merits of each approach, with some automakers advocating for a hybrid system that combines the strengths of both standards.Edge computing in smart traffic infrastructure
Edge computing plays a crucial role in realizing the full potential of V2X communication. By processing data closer to its source, edge computing reduces latency and enables faster decision-making in time-sensitive scenarios. Smart traffic lights equipped with edge processors can dynamically adjust signal timing based on real-time traffic conditions, optimizing flow and reducing congestion. This decentralized approach to traffic management enhances the overall efficiency and responsiveness of autonomous transportation networks.Blockchain for secure data exchange in connected vehicles
As vehicles become increasingly connected, ensuring the security and integrity of data exchange becomes paramount. Blockchain technology offers a promising solution for creating a tamper-resistant, decentralized ledger of vehicle-to-vehicle and vehicle-to-infrastructure communications. By employing cryptographic techniques, blockchain can help prevent spoofing attacks and ensure the authenticity of critical safety messages, building trust in the V2X ecosystem.Ethical and legal frameworks governing autonomous driving
The advent of autonomous vehicles raises complex ethical and legal questions that society must grapple with as this technology becomes more prevalent on our roads. Establishing clear guidelines and regulations is essential to ensure the safe and responsible deployment of self-driving cars.Trolley problem scenarios in AI decision-making
One of the most challenging ethical dilemmas in autonomous driving is how to program vehicles to make decisions in unavoidable accident scenarios. The classic "trolley problem" takes on new significance when applied to self-driving cars: should a vehicle prioritize the safety of its occupants or minimize overall harm, even if it means putting its passengers at risk? Automakers and policymakers must work together to develop ethical frameworks that balance safety, fairness, and societal values in these edge cases.Liability distribution in autonomous vehicle accidents
As control shifts from human drivers to AI systems, traditional notions of liability in automotive accidents must be reevaluated. Questions arise about who bears responsibility in the event of a crash involving an autonomous vehicle: the manufacturer, the software developer, or the vehicle owner? Some jurisdictions are exploring new insurance models and legal frameworks to address these complexities, including the concept of "electronic personhood" for AI systems.Data privacy regulations for connected car networks
The vast amount of data generated and shared by autonomous and connected vehicles raises significant privacy concerns. Regulations like the European Union's General Data Protection Regulation (GDPR) set standards for data collection, storage, and usage in connected car networks. Balancing the need for data-driven improvements in safety and efficiency with individual privacy rights remains an ongoing challenge for legislators and industry stakeholders.Impact of autonomous vehicles on urban planning and infrastructure
The widespread adoption of autonomous vehicles is set to transform not only how we travel but also the very fabric of our cities and transportation infrastructure. Urban planners and policymakers must anticipate and adapt to these changes to create more efficient, sustainable, and livable urban environments.Redesigning road systems for mixed autonomous and human-driven traffic
As autonomous vehicles begin to share the road with human-driven cars, new approaches to road design and traffic management will be necessary. Dedicated lanes for self-driving vehicles, smart intersections that optimize traffic flow based on real-time data, and adaptive road markings that can communicate with autonomous systems are just a few examples of how infrastructure may evolve. The challenge lies in creating a flexible system that can accommodate both autonomous and human-driven vehicles during the transition period.Smart city integration with autonomous transportation networks
The integration of autonomous vehicles into smart city ecosystems presents opportunities for unprecedented levels of urban efficiency and sustainability. Connected transportation networks can work in harmony with other city systems, such as energy grids and waste management, to optimize resource usage and reduce environmental impact. For example, autonomous electric vehicles could serve as mobile energy storage units, helping to balance the power grid during peak demand periods.Parking infrastructure optimization through self-parking systems
Self-parking capabilities in autonomous vehicles have the potential to revolutionize urban parking infrastructure. By eliminating the need for human-friendly spacing and access, parking structures can be redesigned for maximum efficiency, potentially reducing the overall footprint of parking in cities by up to 60%. This freed-up space could be repurposed for housing, green areas, or other community needs, contributing to more livable and sustainable urban environments.Economic and social implications of widespread autonomous adoption
The transition to autonomous vehicles will have far-reaching economic and social consequences, reshaping industries and altering the fabric of society in profound ways. Understanding and preparing for these changes is crucial for policymakers, businesses, and individuals alike.Disruption in the transportation and logistics industry
The advent of autonomous vehicles is set to revolutionize the transportation and logistics sector. Long-haul trucking, one of the largest employers in many countries, could see significant job displacement as self-driving trucks become commercially viable. However, new opportunities may emerge in areas such as fleet management, remote vehicle operation, and maintenance of autonomous systems. The potential for 24/7 operation and increased efficiency could lead to substantial cost savings and productivity gains in the logistics industry.Accessibility improvements for mobility-impaired individuals
Autonomous vehicles have the potential to dramatically improve mobility and independence for individuals with disabilities, the elderly, and those unable to drive conventional vehicles. Self-driving cars could provide on-demand transportation services, expanding access to employment, healthcare, and social activities for millions of people. This increased mobility could lead to greater social inclusion and economic participation for previously underserved populations.Shifts in insurance models for self-driving vehicles
The insurance industry will need to adapt to the changing risk landscape brought about by autonomous vehicles. As human error is eliminated from most driving scenarios, the focus of auto insurance may shift from individual driver policies to product liability coverage for vehicle manufacturers and software providers. Usage-based insurance models that take into account the specific capabilities and safety features of autonomous vehicles are likely to become more prevalent, potentially leading to reduced premiums for consumers.The transition to autonomous vehicles represents one of the most significant shifts in transportation since the invention of the automobile itself. As we navigate this complex landscape, it is crucial to balance technological innovation with careful consideration of its ethical, legal, and societal implications.As autonomous driving technology continues to evolve, its potential to enhance road safety and efficiency becomes increasingly apparent. Through advanced machine learning algorithms, sophisticated sensor technologies, and innovative communication protocols, self-driving cars are poised to dramatically reduce accidents, optimize traffic flow, and transform our urban landscapes. However, realizing these benefits will require thoughtful regulation, ethical decision-making, and adaptive urban planning. The journey towards fully autonomous roads is complex, but the destination promises a safer, more efficient, and more accessible transportation future for all.